基于python的数学建模---时间序列
JetRail高铁乘客量预测——7种时间序列方法
数据获取:获得2012-2014两年每小时乘客数量
import pandas as pd
import numpy as np
import matplotlib.pyplot as plt df = pd.read_csv('C:\\Users\\Style\\Desktop\\jetrail.csv', nrows=11856)
df.head()
print(df.head())
从2012年8月—2013年12月的数据中构造一个数据集
创建train and test文件用于建模。前14个月(2012年8月—2013年10月)用作训练数据,后两个月(2013年11月—2013年12月)用作测试数据。
以每天为单位聚合数据集
import pandas as pd
import matplotlib.pyplot as plt df = pd.read_csv('../profile/train2.csv',nrows=11856) train = df[0:10392] # 前14个月 一共10392个小时
test = df[10392:]
#上表中的 datatime
df['Timestamp'] = pd.to_datetime(df['Datetime'], format='%d-%m-%Y %H:%M') # 4位年用Y,2位年用y
df.index = df['Timestamp']
df = df.resample('D').mean() #按日历采样,计算均值 train['Timestamp'] = pd.to_datetime(train['Datetime'], format='%d-%m-%Y %H:%M')
train.index = train['Timestamp']
train = train.resample('D').mean() test['Timestamp'] = pd.to_datetime(test['Datetime'], format='%d-%m-%Y %H:%M')
test.index = test['Timestamp']
test = test.resample('D').mean() train.Count.plot(figsize=(15,8), title= 'Daily Ridership', fontsize=14)
test.Count.plot(figsize=(15,8), title= 'Daily Ridership', fontsize=14)
plt.show()
结果如下 大致成上升趋势
1.1 朴素法
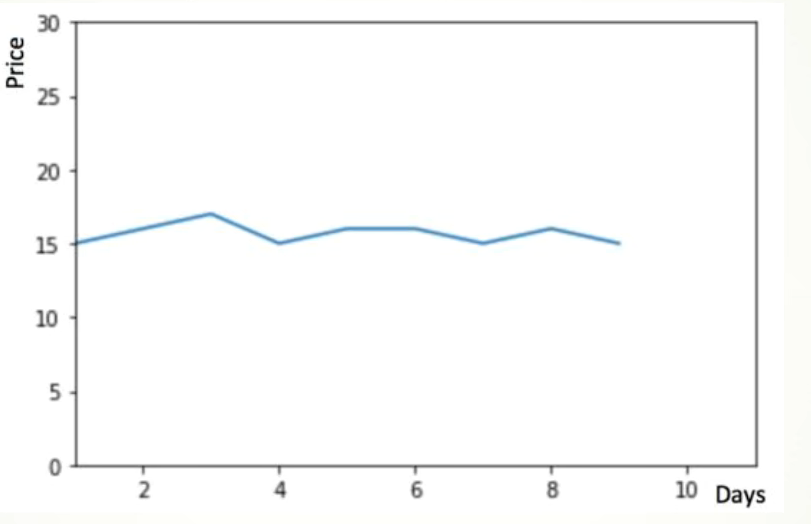
如果数据集在一段时间内都很稳定,我们想预测第二天的价格,可以取前面一天的价格,预测第二天的值。这种假设第一个预测点和上一个观察点相等的预测方法就叫朴素法。
dd = np.asarray(train['Count'])
y_hat = test.copy()
y_hat['naive'] = dd[len(dd) - 1]
plt.figure(figsize=(12, 8))
plt.plot(train.index, train['Count'], label='Train')
plt.plot(test.index, test['Count'], label='Test')
plt.plot(y_hat.index, y_hat['naive'], label='Naive Forecast')
plt.legend(loc='best')
plt.title("Naive Forecast")
plt.show()
最终均方根误差
from sklearn.metrics import mean_squared_error
from math import sqrt rms = sqrt(mean_squared_error(test['Count'], y_hat['naive'])) # 真实的Y和预测的Y值
print(rms)
43.91640614391676
1.2 简单平均法
我们经常会遇到一些数据集,虽然在一定时期内出现小幅变动,但每个时间段的平均值确实保持不变。这种情况下,我们可以预测出第二天的价格大致和过去天数的价格平均值一致。这种将预期值等同于之前所有观测点的平均值的预测方法就叫简单平均法。
y_hat_avg = test.copy()
y_hat_avg['avg_forecast'] = train['Count'].mean()
plt.figure(figsize=(12,8))
plt.plot(train['Count'], label='Train')
plt.plot(test['Count'], label='Test')
plt.plot(y_hat_avg['avg_forecast'], label='Average Forecast')
plt.legend(loc='best')
plt.show()
最终均方根误差
from sklearn.metrics import mean_squared_error
from math import sqrt
rms = sqrt(mean_squared_error(test['Count'], y_hat_avg['avg_forecast']))
print(rms)
109.88526527082863
1.3 移动平均法
物品价格在一段时间内大幅上涨,但后来又趋于平稳。我们也经常会遇到这种数据集,比如价格或销售额某段时间大幅上升或下降。
y_hat_avg = test.copy()
y_hat_avg['moving_avg_forecast'] = train['Count'].rolling(60).mean().iloc[-1]
plt.figure(figsize=(16,8))
plt.plot(train['Count'], label='Train')
plt.plot(test['Count'], label='Test')
plt.plot(y_hat_avg['moving_avg_forecast'], label='Moving Average Forecast')
plt.legend(loc='best')
plt.show()
最终均方根误差
from sklearn.metrics import mean_squared_error
from math import sqrt
rms = sqrt(mean_squared_error(test['Count'], y_hat_avg['moving_avg_forecast']))
print(rms)
46.72840725106963
1.4 简单指数平滑法(之后效果更佳)
from statsmodels.tsa.api import SimpleExpSmoothing y_hat_avg = test.copy()
fit = SimpleExpSmoothing(np.asarray(train['Count'])).fit(smoothing_level=0.6, optimized=False)
y_hat_avg['SES'] = fit.forecast(len(test))
plt.figure(figsize=(16, 8))
plt.plot(train['Count'], label='Train')
plt.plot(test['Count'], label='Test')
plt.plot(y_hat_avg['SES'], label='SES')
plt.legend(loc='best')
plt.show()
最终均方根误差
from sklearn.metrics import mean_squared_error
from math import sqrt rms = sqrt(mean_squared_error(test['Count'], y_hat_avg['SES']))
print(rms)
43.357625225228155
1.5 霍尔特线性趋势法
每个时序数据集可以分解为相应的几个部分:趋势(Trend),季节性(Seasonal)和残差(Residual)。任何呈现某种趋势的数据集都可以用霍尔特线性趋势法用于预测。
import statsmodels.api as sm sm.tsa.seasonal_decompose(train['Count']).plot()
result = sm.tsa.stattools.adfuller(train['Count'])
plt.show()
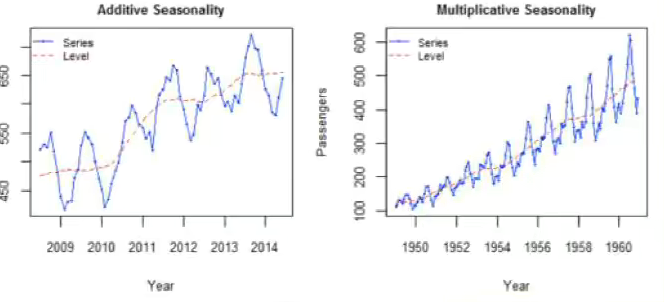
from statsmodels.tsa.api import Holt y_hat_avg = test.copy() fit = Holt(np.asarray(train['Count'])).fit(smoothing_level=0.3, smoothing_slope=0.1)
y_hat_avg['Holt_linear'] = fit.forecast(len(test)) plt.figure(figsize=(16, 8))
plt.plot(train['Count'], label='Train')
plt.plot(test['Count'], label='Test')
plt.plot(y_hat_avg['Holt_linear'], label='Holt_linear')
plt.legend(loc='best')
plt.show()
最终均方根误差
from sklearn.metrics import mean_squared_error
from math import sqrt rms = sqrt(mean_squared_error(test['Count'], y_hat_avg['Holt_linear']))
print(rms)
43.056259611507286
1.6 Holt-Winters季节性预测模型
from statsmodels.tsa.api import ExponentialSmoothing y_hat_avg = test.copy()
fit1 = ExponentialSmoothing(np.asarray(train['Count']), seasonal_periods=7, trend='add', seasonal='add', ).fit()
y_hat_avg['Holt_Winter'] = fit1.forecast(len(test))
plt.figure(figsize=(16, 8))
plt.plot(train['Count'], label='Train')
plt.plot(test['Count'], label='Test')
plt.plot(y_hat_avg['Holt_Winter'], label='Holt_Winter')
plt.legend(loc='best')
plt.show()
最终均方根误差
from sklearn.metrics import mean_squared_error
from math import sqrt rms = sqrt(mean_squared_error(test['Count'], y_hat_avg['Holt_Winter']))
print(rms)
25.264160714051183
1.7 自回归移动平均模型(ARIMA)
指数平滑模型都是基于数据中的趋势和季节性的描述,而自回归移动平均模型的目标是描述数据中彼此之间的关系。ARIMA的一个优化版就是季节性ARIMA。它像Holt-Winters季节性预测模型一样,也把数据集的季节性考虑在内。
import statsmodels.api as sm y_hat_avg = test.copy()
fit1 = sm.tsa.statespace.SARIMAX(train.Count, order=(2, 1, 4), seasonal_order=(0, 1, 1, 7)).fit()
y_hat_avg['SARIMA'] = fit1.predict(start="2013-11-1", end="2013-12-31", dynamic=True)
plt.figure(figsize=(16, 8))
plt.plot(train['Count'], label='Train')
plt.plot(test['Count'], label='Test')
plt.plot(y_hat_avg['SARIMA'], label='SARIMA')
plt.legend(loc='best')
plt.show()
最终均方根误差
from sklearn.metrics import mean_squared_error
from math import sqrt rms = sqrt(mean_squared_error(test['Count'], y_hat_avg['SARIMA']))
print(rms)
26.069547371326845
最新文章
- UML类图关系--继承(泛化)、实现、关联、聚合、组合、依赖
- 【C语言】二维指针做形参
- javascript photo http://www.cnblogs.com/5ishare/tag/javascript/
- 剑指offer系列49--求1+2+...+N的和
- 【HDOJ】3553 Just a String
- 编码神器之sublime(插件安装)
- HDU 5044 TREE
- JSONP技术原理及实现
- javascript的prototype原理理解
- BNUOJ29065鸣人的查克拉
- C++标准库类型vector及迭代器iterator简介
- win10+ubuntu双系统安装方案
- Java基于opencv—透视变换矫正图像
- [UE4]碰撞机制
- Leetcode 784
- idea中使用Mybatis plugin
- ubuntu16.0.4安装mysql5.7以及设置远程访问
- ES6—— 变量的结构赋值
- MySQL数据库实验四:嵌套查询
- 利用xsltproc转换jtl报告到html报告