Apache Kafka for Item Setup
At Walmart.com in the U.S. and at Walmart’s 11 other websites around the world, we provide seamless shopping experience where products are sold by:
- Own Merchants for Walmart.com & Walmart Stores
- Suppliers for Online & Stores
- Sellers on Walmart’s marketplaces
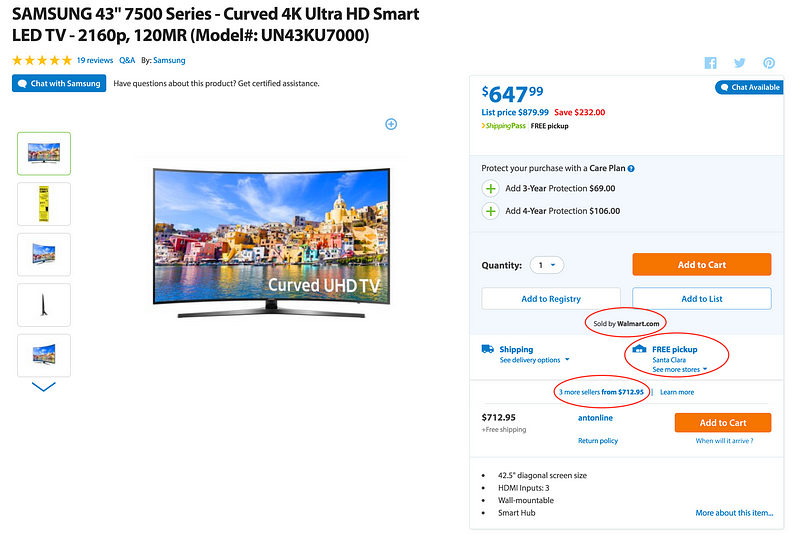
Product sold on walmart.com - Online, Stores by Walmart & by 3 marketplace sellers
The Process is referred to internally as “Item Setup” and the visitors to the sites see Product listings after data processing for Products, Offers, Price,Inventory & Logistics. These entities are comprised of data from multiple sources in different formats & schemas. They have different characteristics around data processing:
- Products requires more of data preparation around:
- Normalization — This is standardization of attributes & values, aids in search and discovery
- Matching — This is a slightly complex problem to match duplicates with imperfect data
- Classification — This involves classification against Categories & Taxonomies
- Content — This involves scoring data quality on attributes like Title, Description, Specifications etc. , finding & filling the “gaps” through entity extraction techniques
- Images — This involves selecting best resolution, deriving attributes, detecting watermark
- Grouping — This involves matching, grouping products based on variations, like shoes varying on Colors & Sizes
- Merging — This involves selection of the best sources and data aggregation from multiple sources
- Reprocessing — The Catalog needs to be reprocessed to pickup daily changes
2. Offers are made by Multiple sellers for same products & need to checked for correctness on:
- Identifiers
- Price variance
- Shipping
- Quantity
- Condition
- Start & End Dates
3. Pricing & Inventory adjustments many times of the day which need to be processed with very low latency & strict time constraints
4. Logistics has a strong requirement around data correctness to optimize cost & delivery
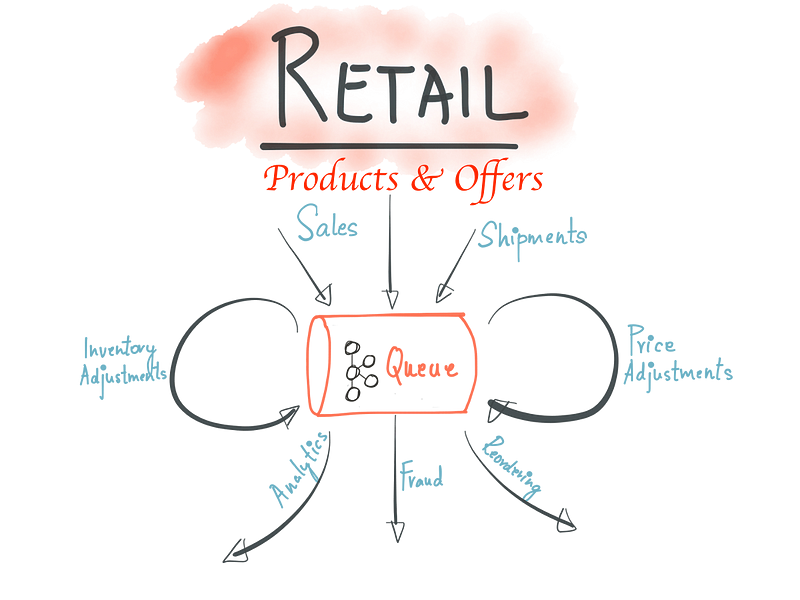
Modified Original with permission from Neha Narkhede
This yields architecturally to lots of decentralized autonomous services, systems & teams which handle the data “Before & After” listing on the site. As part of redesign around 2014 we started looking into building scalable data processing systems. I was personally influenced by this famous blog post “The Log: What every software engineer should know about real-time data’s unifying abstraction” where Kafka could provide good abstraction to connect hundreds of Microservices, Teams, and evolve to company-wide multi-tenant data hub. We started modeling changes as event streams recorded in Kafka before processing. The data processing is performed using a variety of technologies like:
- Stream Processing using Apache Storm, Apache Spark
- Plain Java Program
- Reactive Micro services
- Akka Streams
The new data pipelines which was rolled out in phases since 2015 has enabled business growth where we are on boarding sellers quicker, setting up product listings faster. Kafka is also the backbone for our New Near Real Time (NRT) Search Index, where changes are reflected on the site in seconds.
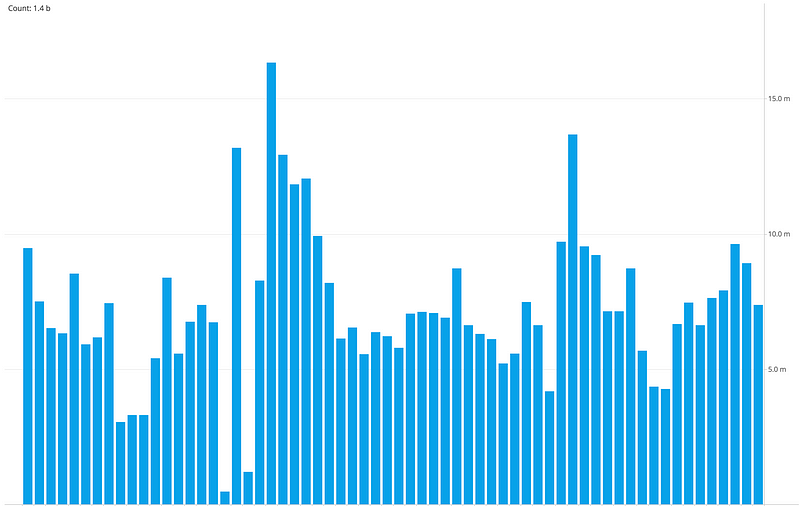
Message Rate filtered for a Day, split Hourly
The usage of Kafka continues to grow with new topics added everyday, we have many small clusters with hundreds of topics, processing billions of updates per day mostly driven by Pricing & Inventory adjustments. We built operational tools for tracking flows, SLA metrics, message send/receive latencies for producers and consumers, alerting on backlogs, latency and throughput. The nice thing of capturing all the updates in Kafka is that we can process the same data for Reprocessing of the catalog, sharing data between environments, A/B Testing, Analytics & Data warehouse.
The shift to Kafka enabled fast processing but has also introduced new challenges like managing many service topologies & their data dependencies, schema management for thousands of attributes, multi-DC data balancing, and shielding consumer sites from changes which may impact business.
The core tenant which drove Kafka adoption where “Item Setup” teams in different geographical locations can operate autonomously has definitely enabled agile development. I have personally witnessed this over the last couple of years since introduction. The next steps are to increase awareness of Kafka internally for New & (Re)architecting existing data processing applications, and evaluate exciting new streaming technologies like Kafka Streams and Apache Flink. We will also engage with the Kafka open source community and the surrounding ecosystem to make contributions.
最新文章
- 微信小程序与传统APP十大优劣对比
- 常用前端框架Angular和React的一些认识
- asp.net 微信企业号办公系统-流程设计--流程步骤设置-事件设置
- vnc里鼠标拖动终端就会产生ctrl+c终端
- Java网络编程(模拟浏览器访问Tomcat服务器)
- Linux下安装Android的adb驱动-解决不能识别的问题
- linux常见笔试题
- 开发servlet三种方式
- getElementById 用法的一个技巧
- #Java学习之路——基础阶段二(第二篇)
- 封装继承多态(OOP)
- hadoop1.2.1的安装
- 在浏览器中高效使用JavaScript module(模块)
- uiautomator 代码记录 : BT发送测试
- JDBC编程之预编译SQL与防注入式攻击以及PreparedStatement的使用教程
- sql查询分析器中显示行号
- Autovacuum 的运行限制
- CentOS6下yum升级安装mysql
- python获取参数
- bootstrap Table的使用方法